It’s now understood and accepted that machine learning and AI in general are showing massive potential within a variety of industries. Everything from home comfort, to shipping and logistics, to building smart-cities is being reconsidered in the context of the budding age of artificial intelligence. Among all the changes though, some of the most exciting and impactful ones are coming in the healthcare industry, with the rapid development in healthtech.
Even within this category though, the subject of machine learning integration could be broken down into numerous areas of focus. Broadly speaking, the following are some of the most interesting ways in which modern machine learning can be put to use in healthcare:
Automated Research
It’s difficult to overstate just how vast the world of healthcare is when you consider disease research and treatments. Universities, labs, and other care facilities around the world are constantly studying diseases and working on new innovations for the sake of prevention and healing. Connecting all of this research can lead to faster and more meaningful progress, and is one of the major challenges the healthcare industry faces today.
This, however, is where automated research can come into play. We have talked in the past about Thorgate working to establish automated research analysis alongside HealX, a Cambridge (UK) based health tech startup. The initiative is specifically focused on setting up a machine-driven data-collection application that can sift through existing drugs and analyse their potential effectiveness in treating new diseases. This is merely one example, but it demonstrates how intelligent systems can be used to speed up and perfect vital research in healthcare. To read in detail about what Thorgate did for Healx, see our case study here.
Automated Communication
Communication within healthcare entails both internal communication between healthcare institutes as well as communication with patients. Thorgate recently helped in developing TXP Chat, a secure mobile messaging application by OmniLife which is uniquely designed for the decentralised and complex communication inherent in organ referrals, offerings, allocation, procurement and transplantation. While machine learning plays a vital role in internal communication through apps such as TXP chat, it is also extremely useful for external communication with patients traditionally known as Marketing.
Marketing is mainly not considered a major factor influencing the healthcare industry, but rest assured it plays a vital role in the industry. While it may not directly impact patient care or disease research, marketing efforts help hospitals, doctors’ offices, prescription drug companies, and pharmacies present themselves to the public. It is largely thanks to continual branding and advertising efforts that people know which names to trust, or where to look first when seeking a given treatment. Especially with the rise of tele-medicine many private healthcare advisors are able to not only market their services online but also provide to their patients.
Today, this side of the industry can also be made more effective thanks to automated services. While marketing tends to require a human touch, Ayima explains how technology can be applied to modern efforts, blending with talent to create a more comprehensive approach. Basically, machine learning can now be used in the gathering and analysis of data that can help provide any given company with an exact direction and strategy for its marketing efforts. In healthcare, this kind of approach can mean that technology enables a pharmacy, hospital, or drug provider to better reach the patients most likely to need assistance.
Advanced Diagnostics
The ability to diagnose conditions quickly and accurately is really at the heart of the healthcare industry. Fortunately, it’s another aspect of the said industry that is beginning to benefit significantly from machine learning developments and adoption.
Wired identifies the healthcare industry’s massive pool of data as what makes this possible. In the current scenario, relevant companies maintain incredible amounts of information about past patients and cases, all of which can be put to use in diagnosing new patients. Essentially, if a patient presents concerns or elaborates symptoms, the details of his or her case can be plugged into a system that, through machine learning, recognises similar cases among past patients. This can result in quick, accurate diagnosis, and the rapid presentation of effective care options.
Robotic Surgery
Surgery is also a fascinating area to consider — and perhaps the one in which machine learning applications seem most like something out of science fiction. To some extent, machine learning can improve surgery in the planning and conversation phases. This is detailed by Technology Networks, which explains that before actually performing procedures, doctors tend to go over different methods, plan their incisions, and discuss options and risks with patients. During these processes, machine learning networks can help to assess possibilities and present low-risk solutions, not entirely dissimilar to how they can analyse cases and suggest treatments.
Additionally though, we may soon be seeing more surgery that is actually performed by robotic systems. Such systems can already perform some procedures, and as they continue to “learn” and develop more, they will almost undoubtedly take on more complex surgeries — and do so with greater precision and reliability than human counterparts.
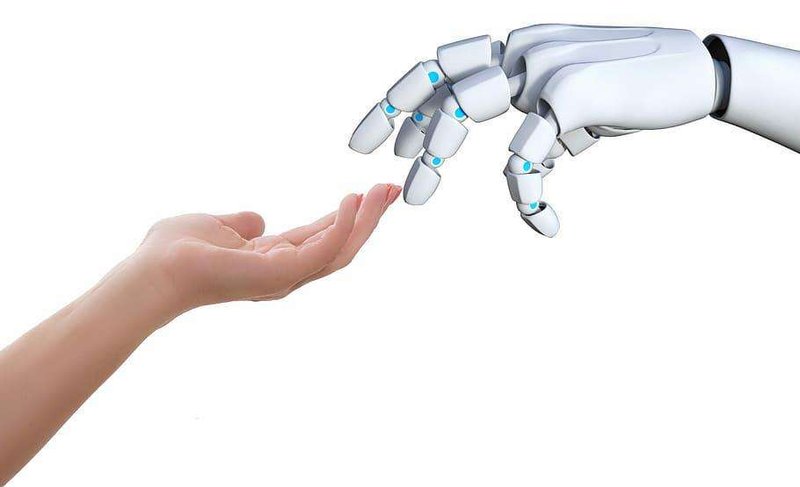
Conclusion
These are few examples to illustrate the breadth of machine learning’s impact on healthcare. The technology is beginning to play a role in everything from marketing and research to surgery and diagnostics, and at this stage it’s fair to say that it’s only just getting started.
This guest post is contributed by Jennylyn Briston.
Read related articles:
Key Takeaways from EHiN 2019 and the Trends in Healthtech